Microsoft's Planetary Computer vs Google Earth Engine: Shaping the Future of Environmental Insight
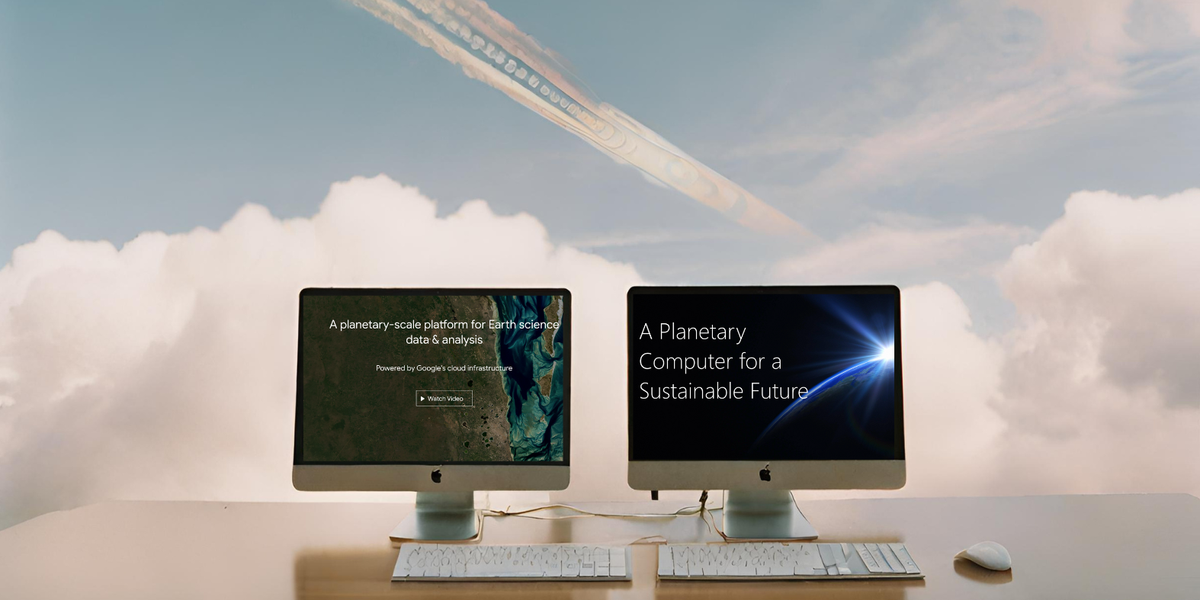
Microsoft's Planetary Computer and Google Earth Engine are leading platforms for enabling a data-driven approach to developing sustainable solutions for environmental challenges. Let's explore these platforms' features, data sources, potential algorithms, and collaboration possibilities.
Microsoft's Planetary Computer: A Holistic Data Repository
Microsoft's Planetary Computer is a holistic data repository. It captures a vast amount of environmental data, including satellite imagery that records land use, vegetation cover, and landscape change. It assimilates data from sensors that measure temperature, air and water quality, climate, biodiversity, land use, human activity, and geospatial data. This comprehensive repository aims to provide a holistic understanding of environmental dynamics on a global scale. Focusing on a holistic understanding of environmental dynamics implies looking at the bigger picture by considering how different elements of an ecosystem interact and influence each other. For example, restoring a wetland means not just focusing on water quality, but also considering how changes will affect the whole ecosystem: the plants, animals, soil and how they relate to each other. This comprehensive view helps to make more informed decisions for effective ecosystem management and restoration.
Google Earth Engine: Rich Archives of Historical Data
In contrast, Google Earth Engine capitalizes on a vast repository of historical satellite imagery and climate datasets. Its historical archives enable detailed analysis and comparisons spanning extended periods. The platform's strength lies in its ability to access and process extensive historical environmental data, facilitating long-term trend analysis and in-depth historical assessments.
AI-Driven Insights and Solutions
Both platforms rely on machine learning and AI algorithms to interpret their massive datasets. While Microsoft's Planetary Computer does not provide specific details about the algorithms built directly into the platform, it does provide examples of machine learning and artificial intelligence algorithms commonly used on similar holistic datasets:
- Image Recognition and Classification: Machine learning algorithms for image recognition can analyse satellite imagery to identify changes in land cover, track deforestation, monitor urban expansion, or detect changes in natural habitats.
- Natural Language Processing (NLP): NLP algorithms can process textual data, including scientific papers, reports, or environmental surveys, to extract relevant information, sentiment analysis, or trends related to environmental issues.
- Clustering and Pattern Recognition: These algorithms group similar environmental data points together, allowing for the identification of clusters or patterns that might signify ecological changes, such as habitat fragmentation or ecosystem shifts.
- Geospatial Analysis Algorithms: These algorithms handle spatial data, analysing relationships between geographical features and environmental factors. They help in understanding spatial dependencies and identifying potential areas for conservation or intervention.
- Reinforcement Learning: Applied in scenarios where the system learns through interactions, reinforcement learning could be used to optimize decision-making in scenarios like wildlife conservation, where actions and consequences are interlinked.
As Google Earth Engine specialises in historical satellite imagery and time-series climate datasets, the most commonly used algorithms are:
- Predictive Modelling Algorithms: These algorithms forecast future environmental conditions based on historical data, enabling predictions about climate patterns, biodiversity changes, or land use transformations. They can employ techniques like regression, time series analysis, or neural networks.
- Anomaly Detection Algorithms: These algorithms identify unusual or irregular patterns in data, which could signal environmental events like pollution spikes, abnormal weather patterns, or sudden ecological changes.
- Time Series Analysis: These algorithms examine data collected over sequential time intervals to discern patterns, trends, or fluctuations. In ecological studies, time series analysis can reveal long-term changes in environmental parameters, such as temperature variations, precipitation levels, or habitat alterations.
- Spectral Analysis: Studying the frequency components of a signal or dataset. In ecological studies, spectral analysis of remote sensing data, like satellite imagery or spectral reflectance measurements, is valuable. It helps researchers discern information about vegetation health, land cover changes, or biodiversity.
Collaborative Potential
Although they are separate entities, the combination of Microsoft's Planetary Computer and Google Earth Engine could provide a broader and deeper understanding of environmental change. Imagine integrating Google Earth Engine's historical data with the real-time data capabilities of Microsoft's platform. This collaboration could provide a more holistic view, supporting long-term trend analysis and predictive modelling.
Despite their rich data repositories, challenges remain in ensuring interoperability and harmonisation of disparate datasets, especially when they are of this scale. Complexity arises from reconciling diverse sensor technologies, temporal resolutions and geographical sources. However, overcoming these hurdles and developing algorithms on unified data from these two platforms could lead to accurate, comprehensive and actionable environmental insights.